-
Notifications
You must be signed in to change notification settings - Fork 1
/
Copy pathdata_version_control_presentation.qmd
479 lines (368 loc) · 22.6 KB
/
data_version_control_presentation.qmd
1
2
3
4
5
6
7
8
9
10
11
12
13
14
15
16
17
18
19
20
21
22
23
24
25
26
27
28
29
30
31
32
33
34
35
36
37
38
39
40
41
42
43
44
45
46
47
48
49
50
51
52
53
54
55
56
57
58
59
60
61
62
63
64
65
66
67
68
69
70
71
72
73
74
75
76
77
78
79
80
81
82
83
84
85
86
87
88
89
90
91
92
93
94
95
96
97
98
99
100
101
102
103
104
105
106
107
108
109
110
111
112
113
114
115
116
117
118
119
120
121
122
123
124
125
126
127
128
129
130
131
132
133
134
135
136
137
138
139
140
141
142
143
144
145
146
147
148
149
150
151
152
153
154
155
156
157
158
159
160
161
162
163
164
165
166
167
168
169
170
171
172
173
174
175
176
177
178
179
180
181
182
183
184
185
186
187
188
189
190
191
192
193
194
195
196
197
198
199
200
201
202
203
204
205
206
207
208
209
210
211
212
213
214
215
216
217
218
219
220
221
222
223
224
225
226
227
228
229
230
231
232
233
234
235
236
237
238
239
240
241
242
243
244
245
246
247
248
249
250
251
252
253
254
255
256
257
258
259
260
261
262
263
264
265
266
267
268
269
270
271
272
273
274
275
276
277
278
279
280
281
282
283
284
285
286
287
288
289
290
291
292
293
294
295
296
297
298
299
300
301
302
303
304
305
306
307
308
309
310
311
312
313
314
315
316
317
318
319
320
321
322
323
324
325
326
327
328
329
330
331
332
333
334
335
336
337
338
339
340
341
342
343
344
345
346
347
348
349
350
351
352
353
354
355
356
357
358
359
360
361
362
363
364
365
366
367
368
369
370
371
372
373
374
375
376
377
378
379
380
381
382
383
384
385
386
387
388
389
390
391
392
393
394
395
396
397
398
399
400
401
402
403
404
405
406
407
408
409
410
411
412
413
414
415
416
417
418
419
420
421
422
423
424
425
426
427
428
429
430
431
432
433
434
435
436
437
438
439
440
441
442
443
444
445
446
447
448
449
450
451
452
453
454
455
456
457
458
459
460
461
462
463
464
465
466
467
468
469
470
471
472
473
474
475
476
477
478
479
---
title: "Data Version Control"
jupyter: python3
author:
name: Mainye B
url: nyab.notion.com
format:
html:
code-fold: true
theme: darkly
toc: true
number-sections: true
colorlinks: true
---
# What is it?
Data version control is way of making a reproducible journal to replicate your data science workflow. Imagine when you are working with teams everyone has their own way of doing things but how can we make a consensus to have unified way of working together so that you don't step on each others toes. On the other hand, is there a way of managing data science projects a bit easier to be able to track project a bit better? We will discuss that in this presentation.
They are several tools that have been created to address this problem. They include the following:
- [DVC](https://dvc.org/)
- [Mlflow](https://mlflow.org/)
- [Neptuneai](https://neptune.ai/)
- [Delta Lake](https://delta.io/)
- [Metaflow](https://metaflow.org/)
::: callout
We'll go through DVC, and Makefiles. Great Expectations is another tool that can be used to validate data.
:::
# Why is it important?
As professionals who have worked on various projects in data science and machine learning, we have discovered that the path from idea to product needs a frictionless workflow. This allows us to focus on implementing ideas rather than handling all that goes on in the background.
It is important mostly because it can get very confusing when handling projects and keeping track of our experiments. In data science, we don't have predefined outputs. We can create reports, dashboards, applications, and APIs. There are so many things that go into that process, such as data importing, exploratory data analysis, feature engineering, and modeling. Each of these steps can take different routes to reach our destination.
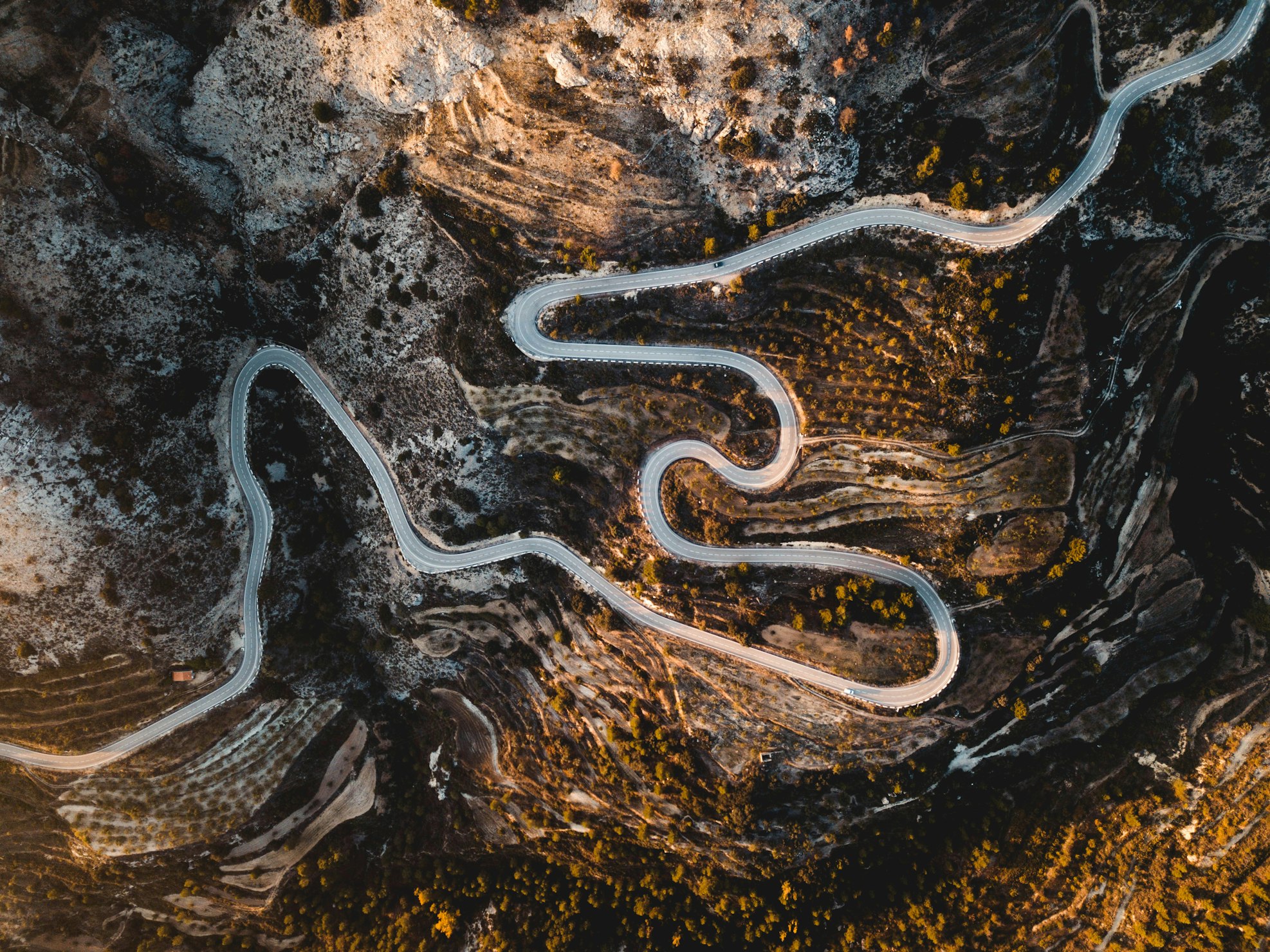{fig-alt="A photo of a winding road"}
## Needs
- How can we track different parts of our work?
- How can we record hyperparameters for different versions of our experiments?
- How can we store metadata of our projects, such as models and slices of data?
- How can we unify and organize metrics?
- Can I fully replicate their work or at least a significant portion of it?
> All of the solutions mentioned above can help address these challenges and can improve your workflows.
### Data Examples
We will be using two datasets for this presentation. The first dataset is the Medical Cost Personal Datasets. This dataset contains information about the medical costs of individuals. The second dataset is the Telco dataset. This dataset contains information about the customers of a telecommunications company. Both datasets are available on Kaggle.
We recommend visiting the [Kaggle website](https://www.kaggle.com/) to download the datasets and explore them further. As well as implement the ideas with the second dataset.
::: callout-important
[**Medical Cost Personal Datasets**](https://www.kaggle.com/datasets/mirichoi0218/insurance)
:::
::: callout-tip
[**Telco dataset**](https://www.kaggle.com/datasets/blastchar/telco-customer-churn)
:::
The dataset has a number of observations and measurements that are crucial for a prediction task, which is finding churn. Churn refers to the likelihood that a client will stop using the telecommunications company. This is particularly relevant if you are looking at the second dataset, the Telco dataset.
Other very common metrics that you can be asked to calculate in the data science team include:
| Metric | Explanation | Associated link |
|--------------------------------|:--------------------------------------------------------------------------------------------------------------------------------------------|--------------------------------------------------------------------------------------------------------------:|
| Hypothesis testing | Making the website better via focus group testing. | [link](https://medium.com/@gajendra.k.s/hypothesis-testing-33aaeeff5336) |
| Conversion rate | time it takes for a client to move from discovery to becoming a paying customer. | [link](https://www.geeksforgeeks.org/conversion-rate-what-is-it-how-to-calculate-it/) |
| Customer life time value (LTV) | how much a client(s) will generate in their lifetime. | [link](https://www.datacamp.com/tutorial/customer-life-time-value) |
| Recommendation systems | how can we sell cross sell our existing products better | [link](https://medium.com/@Karthickk_Rajah/clustering-based-algorithms-in-recommendation-system-205fcb15bc9b) |
| Optimization | adjusting cost of product this involves using specific techniques to find the maximum or minimum value of something to reap better revenues | [link](https://towardsdatascience.com/production-fixed-horizon-planning-with-python-8dd38b468e86) |
### Data science process
We will be referencing a cool notebook that someone in the kaggle community had done. Here's the original [notebook](https://www.kaggle.com/code/hely333/eda-regression).
The person did are really cool job. However, I wish more one hot encoding was done and exploring techniques such as One R were done. We'll explore that later. At the moment, let's set out attention to the data science process.
::: {#fig-datasci layout-ncol="2"}
[](https://www.manning.com/books/data-science-with-python-and-dask)
{width="100"}
What is done in data science
:::
As you can see above we change data in various forms that we can use to understand it better. We can use it to make predictions, make recommendations, and optimize our products.
Often times you can easily just make a notebook, and your work is done. They are tools that allow you to do [scheduled notebook reruns](https://www.kaggle.com/discussions/getting-started/293861) on kaggle, using [papermill](https://papermill.readthedocs.io/en/latest/) and [Sagemaker](https://towardsdatascience.com/how-to-schedule-jupyter-notebooks-in-amazon-sagemaker-d50fa1c8c0ad).
## Try something different with DVC and Makefiles
### Makefile
In most Unix systems (Mac Os and Linux) you'll find that the `make` command is already installed. If not it very easy to install it.
::: callout-tip
How to install
```{bash}
# update packages
sudo apt-get update
# just say yes to make
sudo apt-get -y install make
# what version was installed
make -v
```
:::
Using these files makes it easy to hide the complexity of running commands that you require to follow best practices as an example:
> Running in bash
```{bash}
#| echo: false
# This code runs the pylint tool with specific configurations to check for errors in Python files.
# The `--disable=R,C` flag disables the pylint checks for code style and convention violations.
# The `--errors-only` flag ensures that only error messages are displayed.
# The `*.py utils/*.py testing/*.py` argument specifies the files and directories to be checked by pylint.
pylint --disable=R,C --errors-only *.py utils/*.py testing/*.py
```
Code Linting Linting is crucial for maintaining high-quality code. It helps catch errors and inconsistencies early on, reducing bugs and improving readability.
Why Lint?
- Reduced bugs: Catch errors before runtime.
- Improved readability: Enforce consistent coding standards.
- Faster development: Identify issues quickly.
> Within your Makefile
```{bash}
#| echo: false
lint: activate install format # These are prerequisites: that is they must be run first
# flake8 or #pylint
pylint --disable=R,C --errors-only *.py utils/*.py testing/*.py
```
> In Terminal
```{bash}
#| echo: false
make lint
```
::: callout-tip
Instead of memorizing long commands you can store them in a Makefile and run them in a single command for example `make all` will run each command until the end of the file. Also, Continuous[Integration/Continuous Deployment](https://www.youtube.com/watch?v=2wSBAkJGcug)
:::
### Using a Makefile for Machine Learning Workflow
At this juncture, you are probably acknowledging how much a Makefile is amazing. Get this you can use it with any language you prefer for data science and machine learning. Here are more [examples in Julia and R](https://gist.github.com/Shuyib/ae87774fd82c69706803725db9a681dc)
Let create a Makefile to assist us with **Making** a machine learning workflow to help us handle the project better.
In the directory `datavc_makefile` we have a custom Makefile that we can use to run our commands. Specifically, for a machine learning project.
```{Makefile}
#| echo: false
# .DEFAULT_GOAL tells make which target to run when no target is specified
.DEFAULT_GOAL := all
# .PHONY tells make that these targets do not represent actual files
.PHONY: all install clean format lint create_dirs activate_venv import_data clean_data eda split_data evaluate_model
# run all commands
all: create_dirs install activate_venv import_data clean_data eda split_data evaluate_model
# Specify python location in virtual environment it ensures that the correct version of python is used
# Specify pip location in virtual environment it ensures that the correct version of pip is used
ORIGINAL_PY_VERSION := $(shell python3 --version)
PYTHON := .venv/bin/python3
PIP := .venv/bin/pip3
DOCKER_CONTAINER_NAME := ml_regression_workflow:v0.0.0
DATA_DIR := data/
OUTPUT_DIR := output/
MODEL_OUTPUT_DIR := model_output/
venv/bin/activate: requirements.txt
# create virtual environment
python3 -m venv .venv
# make command executable
chmod +x .venv/bin/activate
# activate virtual environment
. .venv/bin/activate
activate_venv:
# activate virtual environment
# run . .venv/bin/activate manually if it doesn't work
@echo "Activating virtual environment"
chmod +x activate_venv.sh
./activate_venv.sh
install: venv/bin/activate requirements.txt # prerequisite
# install commands
# This is step 1: install the virtual environment
# Py version using py 3.10 from envname
@echo "Python version: $(ORIGINAL_PY_VERSION)"
@echo "Installing virtual environment"
@echo "This is step 1: install the virtual environment"
$(PIP) --no-cache-dir install --upgrade pip &&\
$(PIP) --no-cache-dir install -r requirements.txt
docstring:
# format docstring
pyment -w -o numpydoc *.py
format:
# format code
black *.py
clean:
@echo "Cleaning up"
# clean directory of cache
rm -rf __pycache__ &&\
rm -rf utils/__pycache__ &&\
rm -rf testing/__pycache__ &&\
rm -rf .pytest_cache &&\
rm -rf .venv
rm -rf db
rm -rf data
rm -rf output
rm -rf model_output
lint: activate install format
# flake8 or #pylint
pylint --disable=R,C --errors-only *.py utils/*.py testing/*.py
# Make sure the directories have been created
create_dirs:
@echo "Creating directories"
@echo "This is step 2: create directories"
mkdir -p -v $(DATA_DIR)
mkdir -p -v $(OUTPUT_DIR)
mkdir -p -v $(MODEL_OUTPUT_DIR)
@echo "Directories created"
@echo "remember to follow these steps https://www.kaggle.com/discussions/general/74235"
import_data: create_dirs
@echo "Importing data from Kaggle"
@echo "This is step 3: import data"
@echo "The data folder has a new dataset"
@echo "Your task Can you accurately predict insurance costs? Regression problem"
# make sure script is executable
chmod +x import_data.sh
# run script
./import_data.sh
clean_data: import_data data/original_data/insurance.csv
@echo "Cleaning data"
@echo "This is step 4: clean data"
@echo "The data folder has a cleaned dataset in data/transform"
$(PYTHON) cleandata.py load_data --file_path data/original_data/insurance.csv
$(PYTHON) cleandata.py summary --file_path data/original_data/insurance.csv
$(PYTHON) cleandata.py check_missing --file_path data/original_data/insurance.csv
$(PYTHON) cleandata.py check_duplicate --file_path data/original_data/insurance.csv
$(PYTHON) cleandata.py encode_data --file_path data/original_data/insurance.csv --version 000
@echo "Data cleaned"
eda: clean_data
@echo "Performing EDA"
@echo "This is step 5: EDA"
@echo "The output folder has an EDA report in output/eda"
$(PYTHON) eda.py --input data/transform/insurance_000.parquet --output output/eda_combined_plots.png
split_data: eda
@echo "Splitting data"
@echo "This is step 6: split data"
@echo "The output folder has a split dataset in data/transform/validation"
@echo "For train test split"
$(PYTHON) split_data.py --data data/transform/insurance_000.parquet --strategy train_test_split --test_size 0.2
@echo "For kfold split"
#$(PYTHON) split_data.py --data data/transform/insurance_000.parquet --strategy kfold --test_size 0.2 --n_splits 5
evaluate_model: split_data
@echo "Evaluating model"
@echo "This is step 7: evaluate model"
@echo "The output folder has a model evaluation in output/model_evaluation"
$(PYTHON) evaluate.py --criterion squared_error --min_samples_leaf 10 --max_leaf_nodes 5 --degree 3
docker_build: requirements.txt Dockerfile
@echo "Building docker image"
sudo docker build -t $(DOCKER_CONTAINER_NAME) .
docker_run: docker_build
@echo "Running docker container"
sudo docker run -it --rm $(DOCKER_CONTAINER_NAME)
docker_clean:
@echo "Cleaning up docker"
sudo docker rmi $(DOCKER_CONTAINER_NAME)
```
This Makefile encompasses the whole machine learning workflow. It is a great way to keep track of your work, and also to `Make` sure that you are following best practices. For example, this can encompasses your development, testing, and deployment workflow based on software engineering principles. In addition, the addition of a Dockerfile improves the reproducibility of your work. You can run the commands in the Makefile by running `make all` in the terminal. In case something goes wrong in part of the workflow other parts of the workflow will not run. This helps us isolate any potential issues that may arise, improve reliability and maintainability of the project.
::: callout-tip
\$(PYTHON) is a variable that is used to specify the python version that you want to use. This is important because you may have multiple versions of python installed on your machine. This ensures that the correct version of python is used.
\$(PIP) is a variable that is used to specify the pip version that you want to use. This is important because you may have multiple versions of pip installed on your machine. This ensures that the correct version of pip is used.
It is also convenient that you can specify the \$(DOCKER_CONTAINER_NAME) variable and easily change it for different versions of your project.
:::
That's it for the Makefile. Let's move on to DVC.
### DVC
Is another tool that can help you track your data science projects. Most of the time, it is used independently. But, we thought wouldn't it be awesome if we combined Makefile + DVC. That's what we did, and the gains are tremendous. With DVC, you can version control your data, models, and experiments. It allows you to track changes, collaborate with others, and reproduce your results. By integrating DVC with Makefile, you can automate your data science workflow and ensure that all the necessary steps are executed in the correct order. This combination provides a powerful and efficient way to manage your projects and make them more reproducible.
Here is a simple example of how you can use DVC with Makefile.
```{Makefile}
#| echo: false
# .DEFAULT_GOAL tells make which target to run when no target is specified
.DEFAULT_GOAL := all
# .PHONY tells make that these targets do not represent actual files
.PHONY: all install clean format lint create_dirs activate_venv import_data clean_data eda split_data evaluate_model
# run all commands
all:
dvc repro
# Specify python location in virtual environment
# Specify pip location in virtual environment
ORIGINAL_PY_VERSION := $(shell python3 --version)
PYTHON := .venv/bin/python3
PIP := .venv/bin/pip3
DOCKER_CONTAINER_NAME := ML_workflow:v0.0.0
DATA_DIR := data/
OUTPUT_DIR := output/
MODEL_OUTPUT_DIR := model_output/
venv/bin/activate: requirements.txt
# create virtual environment
python3 -m venv .venv
# make command executable
chmod +x .venv/bin/activate
# activate virtual environment
. .venv/bin/activate
activate_venv:
# activate virtual environment
# run . .venv/bin/activate manually if it doesn't work
@echo "Activating virtual environment"
dvc repro activate_venv
install: venv/bin/activate requirements.txt # prerequisite
# install commands
# This is step 1: install the virtual environment
# Py version using py 3.10 from envname
@echo "Python version: $(ORIGINAL_PY_VERSION)"
@echo "Installing virtual environment"
@echo "This is step 1: install the virtual environment"
$(PIP) --no-cache-dir install --upgrade pip &&\
$(PIP) --no-cache-dir install -r requirements.txt
docstring:
# format docstring
pyment -w -o numpydoc *.py
format:
# format code
black *.py
clean:
@echo "Cleaning up"
# clean directory of cache
rm -rf __pycache__ &&\
rm -rf utils/__pycache__ &&\
rm -rf testing/__pycache__ &&\
rm -rf .pytest_cache &&\
rm -rf .venv
rm -rf db
rm -rf data
rm -rf output
rm -rf model_output
lint: activate install format
# flake8 or #pylint
pylint --disable=R,C --errors-only *.py utils/*.py testing/*.py
init:
@echo "Initializing DVC"
dvc init
# Make sure the directories have been created
create_dirs:
@echo "Creating directories"
@echo "This is step 2: create directories"
dvc repro create_dirs
import_data:
@echo "Importing data from Kaggle"
@echo "This is step 3: import data"
@echo "The data folder has a new dataset"
@echo "Your task Can you accurately predict insurance costs? Regression problem"
dvc repro import_data
clean_data: import_data data/original_data/insurance.csv
@echo "Cleaning data"
@echo "This is step 4: clean data"
@echo "The data folder has a cleaned dataset in data/transform"
dvc repro clean_data
eda:
@echo "Performing EDA"
@echo "This is step 5: EDA"
@echo "The output folder has an EDA report in output/eda"
dvc repro eda
split_data:
@echo "Splitting data"
@echo "This is step 6: split data"
@echo "The output folder has a split dataset in data/transform/validation"
@echo "For train test split"
dvc repro split_data
evaluate_model:
@echo "Evaluating model"
@echo "This is step 7: evaluate model"
@echo "The output folder has a model evaluation in output/model_evaluation"
dvc repro evaluate_model
compare_metrics:
@echo "Comparing metrics"
@echo "This is step 8: compare metrics"
@echo "The output folder has a model evaluation in output/model_evaluation"
dvc metrics diff
hyperparam_diff:
@echo "Comparing hyperparameters"
@echo "This is step 9: compare hyperparameters"
@echo "The output folder has a model evaluation in output/model_evaluation"
dvc params diff
clear_cache:
@echo "Clearing cache"
@echo "This is step 10: clear cache"
@echo "The output folder has a model evaluation in output/model_evaluation"
rm -rf .dvc/cache
docker_build: requirements.txt Dockerfile
@echo "Building docker image"
sudo docker build -t $(DOCKER_CONTAINER_NAME) .
docker_run: docker_build
@echo "Running docker container"
sudo docker run -it --rm $(DOCKER_CONTAINER_NAME)
```
The difference here is that DVC has specific commands they include `dvc init`, `dvc repro`, `dvc metrics diff`, `dvc params diff`, and `rm -rf .dvc/cache`. These commands are used to track changes, compare metrics, compare hyperparameters, and clear the cache respectively. The `dvc repro` command is used to reproduce the results of the workflow. This ensures that the workflow is executed in the correct order and that all the necessary steps are executed. The `dvc metrics diff` command is used to compare the metrics of different experiments. The `dvc params diff` command is used to compare the hyperparameters of different experiments. The `rm -rf .dvc/cache` command is used to clear the cache. This is important since the cache can take up a lot of space and slow down the workflow. By clearing the cache, you can free up space and speed up the workflow.
Furthermore, there's that file `dvc.yaml` that is created when you run `dvc run {parameters}`. This file is used to track the dependencies of the workflow. It specifies the input and output files of each step in the workflow. This ensures that the correct files are used as input and output for each step. This helps to ensure that the workflow is executed in the correct order and that all the necessary steps are executed. The `params.yaml` file is used to track the hyperparameters of the workflow. It specifies the hyperparameters that are used for each step in the workflow. This helps to ensure that the correct hyperparameters are used for each step. Where as, the `.dvc` and `.dvc.lock` files are used to track the changes in the workflow. They specify the files that have been changed and the changes that have been made. This helps to ensure that the workflow is reproducible and that the results can be reproduced.
### Conclusion
In conclusion, combining Makefile and DVC is a powerful way to manage your data science projects. It allows you to automate your workflow, track changes, collaborate with others, and reproduce your results. By using Makefile and DVC together, you can ensure that your projects are more reproducible, reliable, and maintainable. This can help you save time, reduce errors, and improve the quality of your work. So, next time you start a new data science project, consider using Makefile and DVC to manage your workflow. You won't regret it.
::: callout-tip
We recommend visiting the [Makefile ML](datavc_makefile/README.md) & [Makefile & DVC](datavc_full/README.md) files for implementing the ideas we have put across for the Makefile and for DVC.
:::
## References
1.DVC documentation: <https://dvc.org/doc>\
2.DVC YouTube channel: <https://www.youtube.com/playlist?list=PL7WG7YrwYcnDb0qdPl9-KEStsL-3oaEjg>\
3.Pragmatic AI labs: <https://youtu.be/rKRG6oQf-bQ?si=4BzXMhS7owl6uWef>\
4.Kaggle notebook by Dandelion: <https://www.kaggle.com/code/hely333/eda-regression>\
5.Predicting Chronic kidney Disease: <https://github.com/Shuyib/chronic-kidney-disease-kaggle>