This repository has been archived by the owner on Nov 17, 2023. It is now read-only.
[MXNET-107] Add Fused Vanilla RNN and dropout for CPU #11399
Merged
Add this suggestion to a batch that can be applied as a single commit.
This suggestion is invalid because no changes were made to the code.
Suggestions cannot be applied while the pull request is closed.
Suggestions cannot be applied while viewing a subset of changes.
Only one suggestion per line can be applied in a batch.
Add this suggestion to a batch that can be applied as a single commit.
Applying suggestions on deleted lines is not supported.
You must change the existing code in this line in order to create a valid suggestion.
Outdated suggestions cannot be applied.
This suggestion has been applied or marked resolved.
Suggestions cannot be applied from pending reviews.
Suggestions cannot be applied on multi-line comments.
Suggestions cannot be applied while the pull request is queued to merge.
Suggestion cannot be applied right now. Please check back later.
Description
In this PR, it creates Fused Vanilla RNN(tanh/relu) operator and dropout of GRU/LSTM/vRNN for CPU.
@pengzhao-intel, @TaoLv
Feature changes
New features
Unit-test changes
Performance
We have tested performance of FusedRNN and NonFused RNNCell on our local Skylake-8180 with 2 Sockets and 56 cores. Use MKL as blas lib in this performance test.
Test input size is from DS2 default parameters(seq_length = 300, batch_size = 20, input_size = 800, hidden_size = 800).
Layer=1 bidirectional = False
Layer=5 bidirectional = True
Convergency Curve
We have tested Convergency of FusedGRU/LSTM(dropout = 0.5) on our CPU-Skylake-8180 with 2 Sockets and 56 cores and GPU-P100 by using example/rnn/bucketing/cudnn_rnn_bucketing.py
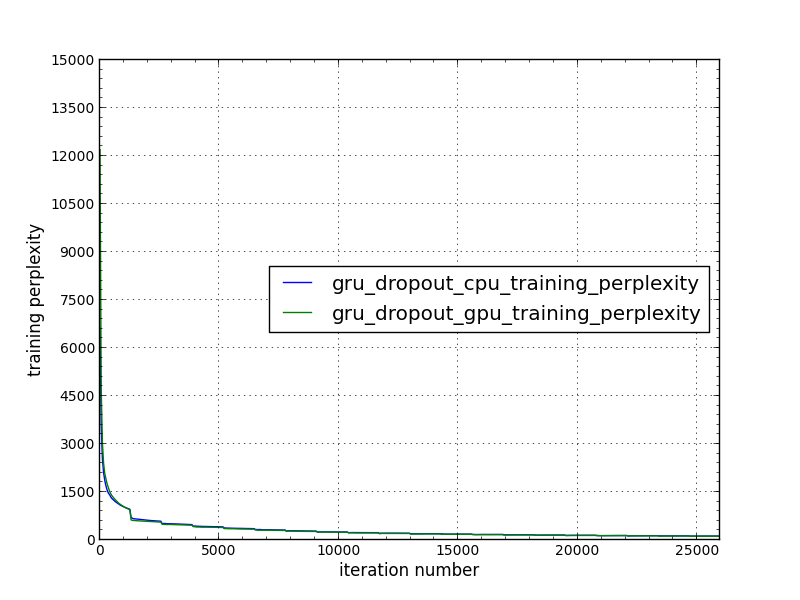
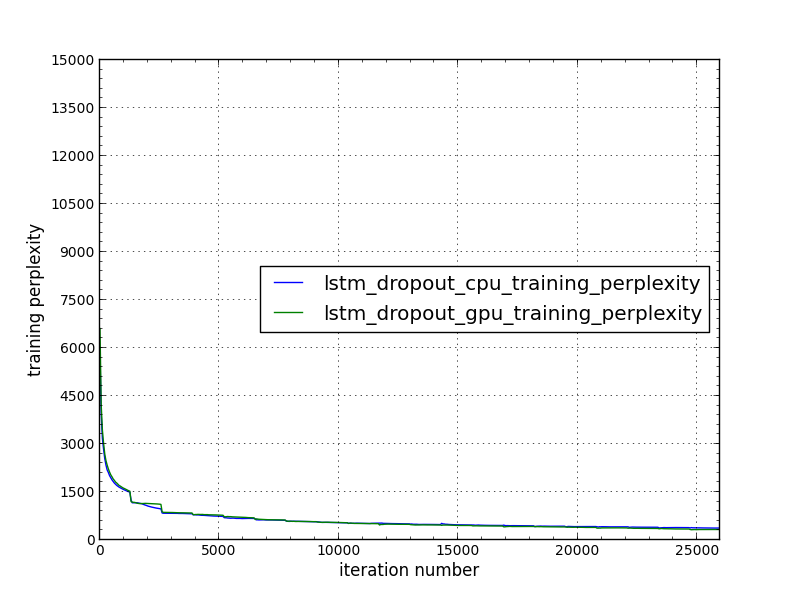
Test input size is layer = 3, batch_size = 32, num-embed = 800, num-hidden = 800, num-epochs 20
@szha: resolves #10870, #10872