You signed in with another tab or window. Reload to refresh your session.You signed out in another tab or window. Reload to refresh your session.You switched accounts on another tab or window. Reload to refresh your session.Dismiss alert
The documentation suggests that the sklearn OneHotEncoder should be a viable transformation when using the MimicExplainer, but I'm getting errors if I use it and set the min_frequency parameter to remove category levels with low counts.
If I set up my data preprocessor like this
(where I have ~7 categorical features, each with many levels)
However, if I set a different transformer for each categorical feature, the Explainer works, albeit with a Many to one/many maps found in input warning and produces outputs that don't really make sense (Half the features end up having very, very similar SHAP values).
The documentation suggests that the sklearn
OneHotEncoder
should be a viable transformation when using theMimicExplainer
, but I'm getting errors if I use it and set themin_frequency
parameter to remove category levels with low counts.If I set up my data preprocessor like this
(where I have ~7 categorical features, each with many levels)
I get the following error
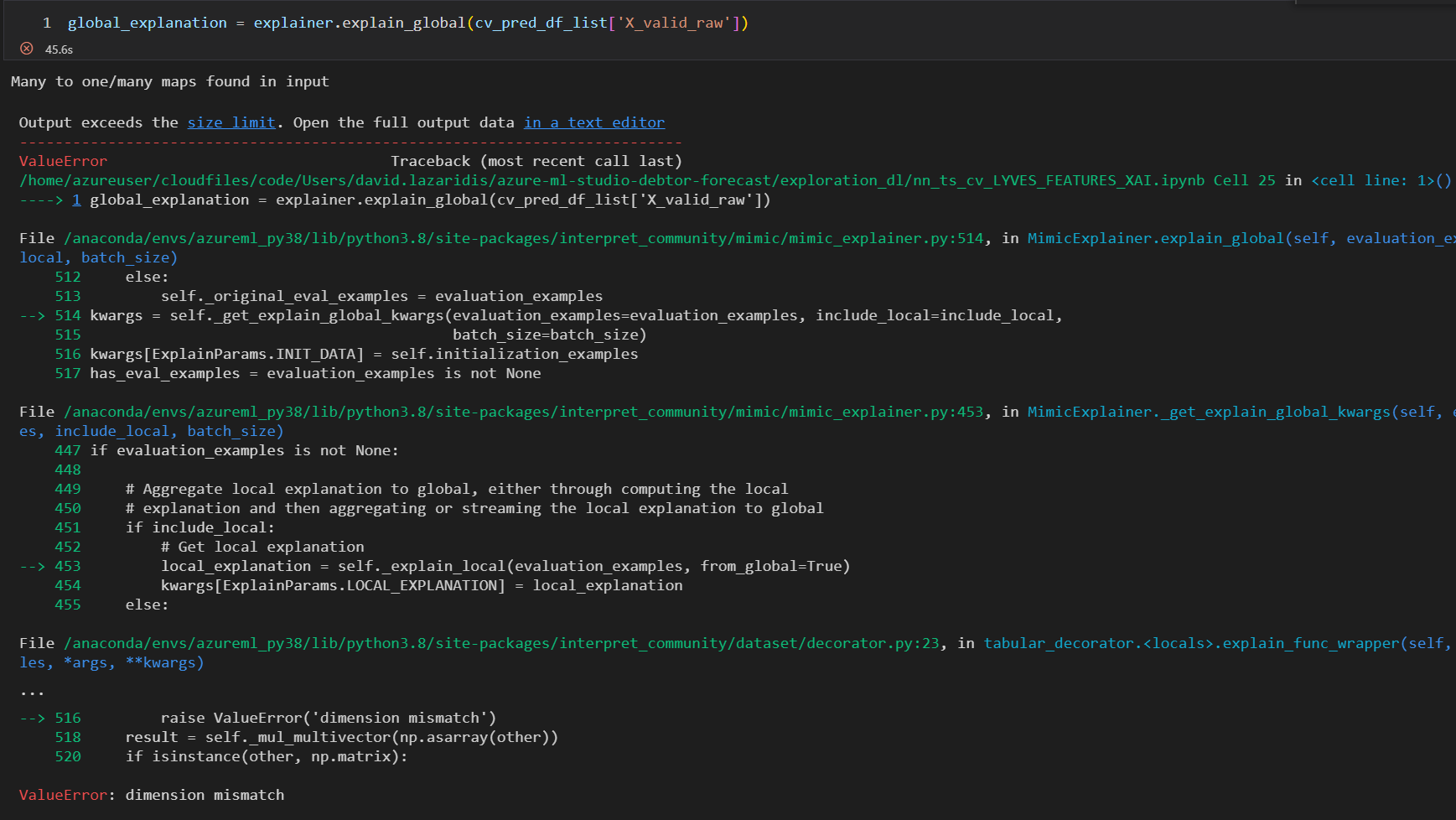
However, if I set a different transformer for each categorical feature, the Explainer works, albeit with a
Many to one/many maps found in input
warning and produces outputs that don't really make sense (Half the features end up having very, very similar SHAP values).The text was updated successfully, but these errors were encountered: