You signed in with another tab or window. Reload to refresh your session.You signed out in another tab or window. Reload to refresh your session.You switched accounts on another tab or window. Reload to refresh your session.Dismiss alert
I use the original LIME to explain some instances of my dataset. I decided to use Interpret's LIME to explain the same instances due to the better user interface we have from Interpret. However, I am explaining the same instance, and using the same conditions the results are different. Any ideas about why the results are not the same?
Here are the results from LIME:
Here are the results from Interpret LIME:
Some thoughts from my side:
1- Is Interpret's LIME using random seed as in LIME or not, if you are not using it then the results will be random from call to call?
2- Is Interpret's LIME by default is doing classification or not?
3- Is Interpret's LIME importing by default the default values set to the different parameters in LimeTabularExplainer and LIME's explain instance or I have to specify them?
This is how I called Interpret's LIME to be exactly under the same conditions as the LIME explainer I am using:
For SHAP kernel explainer, I am not sure if I passed the nsamples parameter used in SHAP during calling the shape values, to the ShapKernel in Interpret's it will work probably or not. I see that It does not make any difference when I pass it or not, so it appears that the class is not importing the parameter correctly.
Third Question:
I know that the ebm model deals with the categorical features automatically is there a way to stop that? I prefer to process my features on my own then fit the model to the processed features directly.
Many thanks.
The text was updated successfully, but these errors were encountered:
Dear All,
First Question:
I use the original LIME to explain some instances of my dataset. I decided to use Interpret's LIME to explain the same instances due to the better user interface we have from Interpret. However, I am explaining the same instance, and using the same conditions the results are different. Any ideas about why the results are not the same?
Here are the results from LIME:
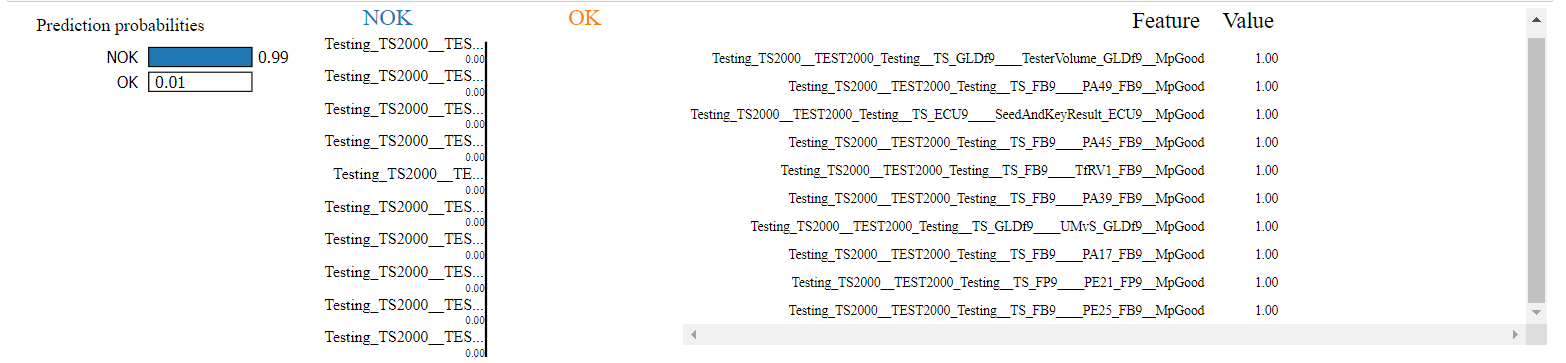
Here are the results from Interpret LIME:
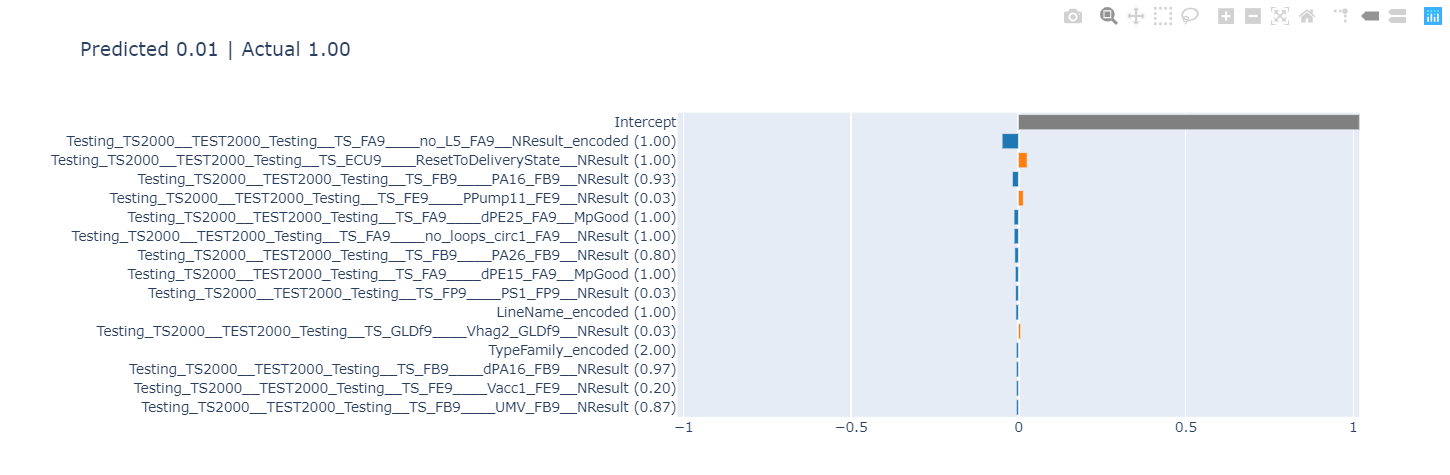
Some thoughts from my side:
1- Is Interpret's LIME using random seed as in LIME or not, if you are not using it then the results will be random from call to call?
2- Is Interpret's LIME by default is doing classification or not?
3- Is Interpret's LIME importing by default the default values set to the different parameters in LimeTabularExplainer and LIME's explain instance or I have to specify them?
This is how I called Interpret's LIME to be exactly under the same conditions as the LIME explainer I am using:
lime_trial = LimeTabular(predict_fn=model.explainer._build_predict_fn(), data=label_encoded_data_train_x, training_labels=label_encoded_data_train_y, categorical_features=model.explainer.categorical_indices, categorical_names=model.explainer.categorical_names, feature_selection='none', feature_names=model.explainer.features_for_lime, discretize_continuous=True, discretizer='quartile', class_names = ['NOK', 'OK'], kernel_width = None, verbose=False, explain_kwargs={'num_features': 10, 'num_samples': 5000, 'top_labels': None, 'distance_metric': 'euclidean', 'model_regressor': None})
lime_trial_xgb = lime_trial.explain_local(label_encoded_interesting_parts_x, label_encoded_interesting_parts_y, name='LIME')
Second Question:
For SHAP kernel explainer, I am not sure if I passed the
nsamples
parameter used in SHAP during calling the shape values, to the ShapKernel in Interpret's it will work probably or not. I see that It does not make any difference when I pass it or not, so it appears that the class is not importing the parameter correctly.Third Question:
I know that the ebm model deals with the categorical features automatically is there a way to stop that? I prefer to process my features on my own then fit the model to the processed features directly.
Many thanks.
The text was updated successfully, but these errors were encountered: