-
Notifications
You must be signed in to change notification settings - Fork 1
/
Copy pathraster.qmd
345 lines (266 loc) · 10.4 KB
/
raster.qmd
1
2
3
4
5
6
7
8
9
10
11
12
13
14
15
16
17
18
19
20
21
22
23
24
25
26
27
28
29
30
31
32
33
34
35
36
37
38
39
40
41
42
43
44
45
46
47
48
49
50
51
52
53
54
55
56
57
58
59
60
61
62
63
64
65
66
67
68
69
70
71
72
73
74
75
76
77
78
79
80
81
82
83
84
85
86
87
88
89
90
91
92
93
94
95
96
97
98
99
100
101
102
103
104
105
106
107
108
109
110
111
112
113
114
115
116
117
118
119
120
121
122
123
124
125
126
127
128
129
130
131
132
133
134
135
136
137
138
139
140
141
142
143
144
145
146
147
148
149
150
151
152
153
154
155
156
157
158
159
160
161
162
163
164
165
166
167
168
169
170
171
172
173
174
175
176
177
178
179
180
181
182
183
184
185
186
187
188
189
190
191
192
193
194
195
196
197
198
199
200
201
202
203
204
205
206
207
208
209
210
211
212
213
214
215
216
217
218
219
220
221
222
223
224
225
226
227
228
229
230
231
232
233
234
235
236
237
238
239
240
241
242
243
244
245
246
247
248
249
250
251
252
253
254
255
256
257
258
259
260
261
262
263
264
265
266
267
268
269
270
271
272
273
274
275
276
277
278
279
280
281
282
283
284
285
286
287
288
289
290
291
292
293
294
295
296
297
298
299
300
301
302
303
304
305
306
307
308
309
310
311
312
313
314
315
316
317
318
319
320
321
322
323
324
325
326
327
328
329
330
331
332
333
334
335
336
337
338
339
340
341
342
343
344
345
# Raster data
As geoscientists, it is very likely that the majority of data we work with is in raster format.
In this section, we will look at how to read raster data into R, how to perform raster/vector operations and basic usage of data cubes.
We need the packages below, where `stars` [@R-stars] and `terra` [@R-terra] are the workforces to handle raster datasets in R.
We load `sf` for raster/vector interaction, `ggplot2`, `tmap` and `units` for visualisation.
```{r}
#| label: libraries
#| warning: false
library(sf) # vector spatial data classes and functions
library(terra) # raster spatial data classes and functions
library(tmap) # noninteractive and interactive maps
library(stars) # spatiotemporal arrays classes and functions
library(ggplot2) # non-spatial and spatial plotting
library(units) # units conversions
```
## Raster-vector operations
We use here datasets from the `spDataLarge` package.
Similar to `spData` this is a data package.
The data consists of terrain derivatives and a set of landslide locations in Ecuador.
The data is used for landslide susceptibility assessment.
We call two vector datasets, `lsl` and `study_mask` first.
We convert `lsl` into an sf object with `sf::st_as_sf()`.
```{r}
data("lsl", "study_mask", package = "spDataLarge")
landslides = st_as_sf(
lsl,
coords = c("x", "y"),
crs = "EPSG:32717"
)
```
As for the raster data, we use `terra::rast()` in this case.
We call the data from its location on file for this using `system.file()`.
```{r}
terrain = rast(system.file("raster/ta.tif", package = "spDataLarge"))
terrain
```
### Masking
Now, the first operation we apply to this raster data is a mask.
We use the `study_mask` object we loaded above to crop our data to a polygon.
```{r}
terrain = mask(terrain, study_mask)
```
Let's take a first glance of our data using `tmap`.
Here we plot the terrain derivatives and the landslide points in separate subplots.
```{r}
#| warning: false
#| message: false
#| fig-width: 15
#| fig-height: 4.5
#| fig-column: page
tm_shape(terrain) +
tm_raster(
col.scale = tm_scale_continuous(midpoint = NA),
col.legend = tm_legend(orientation = "landscape")
) +
tm_facets_hstack() +
tm_shape(landslides) +
tm_dots()
```
### Extraction
Another common operation is to extract raster values at point locations.
We can easily do this using `terra::extract()`.
```{r}
head(terra::extract(terrain, landslides))
```
We did not save the result of this since the landslides object already has the corresponding columns.
```{r}
landslides
```
:::{.callout-tip}
If you use the {tidyverse} and would like to include {terra} to your workflows, take a look at [{tidyterra}](https://dieghernan.github.io/tidyterra/).
The package allows you to plot {terra} objects with {ggplot2} too!
:::
### Predictions
Once we have extracted the data from the terrain derivatives at the landslide point locations,
we can proceed to create a generalized linear model (GLM)
```{r}
fit = glm(lslpts ~ slope + cplan + cprof + elev + log10_carea,
family = binomial(), data = landslides)
```
And next we can use the `terra::predict()` function to apply the model to our terrain data.
```{r}
pred = predict(terrain, model = fit, type = "response")
```
```{r}
#| code-fold: true
tm_shape(pred) +
tm_raster(
col.scale = tm_scale_continuous(midpoint = NA, values = "-viridis"),
col.legend = tm_legend(
title = "",
position = tm_pos_in("left", "bottom"),
width = 10,
frame = FALSE,
orientation = "landscape"
)
) +
tm_title("Landslide susceptibility")
```
::: {.callout-tip}
# Statistical learning
{width=25} For the full example on landslide susceptibility, visit the [Statistical learning chapter](https://r.geocompx.org/spatial-cv.html) in Geocomputation with R.
:::
## Data cubes
Data cubes are data structures that have become popular to organise and analyse raster data in particular.
You may have heard of Earth observation (EO) data cubes.
This are usually data structures that organise satellite imagery and other type of EO data in cubes with multiple dimensions, most specifically the x/y coordinates, time, and bands.
::: {.callout-tip}
# Data cubes
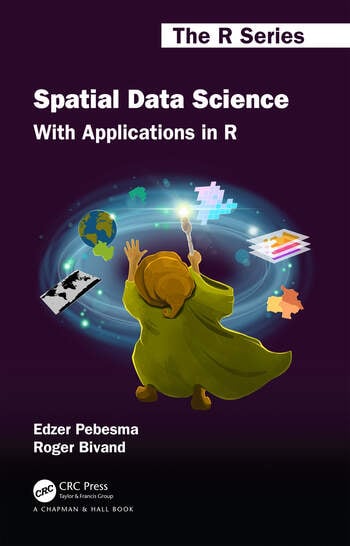{width=25} Learn more about data cubes in the [Data cubes chapter](https://r-spatial.org/book/06-Cubes.html) in Spatial Data Science.
:::
### Satellite imagery
In R, the `stars` package is designed to handle spatiotemporal arrays.
We take a look at their example data, a Landsat-7 scene, read into R using `stars::read_stars()`.
```{r}
tif = system.file("tif/L7_ETMs.tif", package = "stars")
l7 = read_stars(tif)
l7
```
A `stars` object prints out by summarising the attributes at the top and with a dimensions table at the bottom.
In this case we can see the satellite imagery has three dimensions x, y, and band.
```{r}
plot(l7, axes = TRUE)
```
The quick plot above automatically creates subplots of the third dimension of the data, the `band` dimension.
We can subset this dimension using the `[` operator.
```{r}
l7[,,,3:4]
```
And we can create RGB plots with the plot method of stars using the `rgb` parameter to corresponding bands.
```{r}
#| layout-ncol: 2
#| fig-height: 7
plot(l7, rgb = c(3,2,1), reset = FALSE, main = "RGB") # rgb
plot(l7, rgb = c(4,3,2), main = "False colour (NIR-R-G)") # false colour
```
### Applying functions
We can also apply functions over dimensions.
For example, here we create a function to calculate the NDVI.
This function takes two arguments, the red and nir bands.
We then subset the cube with dimensions `3:4`, followed by the dimensions on which this function is applied to and finally the function itself.
```{r}
fn_ndvi = function(red,nir) (nir-red)/(nir+red)
ndvi = st_apply(l7[,,,3:4], 1:2, fn_ndvi)
```
Let's take a look at the result with `tmap`.
```{r}
tm_shape(ndvi) +
tm_raster(
col.scale = tm_scale_continuous(
values = "prgn",
midpoint = 0
)
)
```
::: {.callout-tip}
R has seen a big advance in handling Earth observation data lately.
Packages like [{rstac}](https://brazil-data-cube.github.io/rstac/) and [{gdalcubes}](https://gdalcubes.github.io/) allow the creation of data cubes on-demand to analyse satellite imagery on cloud platforms.
The [SITS](https://e-sensing.github.io/sitsbook/) package and book are great resources to learn about satellite image time series analysis in data cube environments.
:::
### Climate data
One recurrent type of data in the geosciences is climate data.
More generally, gridded datasets that are available at regular time intervals and most often than not distributed as NetCDF files.
This type of data fits perfectly a data cube structure.
We use the `stars` package with its example dataset on precipitation to explore this type of data.
```{r}
precipitation = system.file("nc/test_stageiv_xyt.nc", package = "stars") |>
read_stars()
precipitation
```
This dataset also has three dimensions but instead of band we have time.
To showcase some raster-vector interaction, we load a vector dataset with [North Carolina counties](https://r-spatial.github.io/sf/reference/nc.html).
```{r}
nc = system.file("gpkg/nc.gpkg", package = "sf") |>
read_sf() |>
# Transform CRS to match precipitation data cube
st_transform(st_crs(precipitation))
```
For visualisation purposes, let's create a union of all counties to obtain an outline of North Carolina.
```{r}
nc_outline = nc |>
st_geometry() |>
st_union()
```
Now, using `ggplot2` we can take a look at the precipitation data over time using `ggplot2::geom_stars()` and `ggplot2::facet_wrap()`.
We only plot the first twelve dates.
```{r}
#| fig-width: 10
#| fig-height: 7
ggplot() +
geom_stars(data = precipitation[,,,1:12]) +
scale_fill_viridis_c("Precipitation") +
geom_sf(data = nc_outline, fill = NA, color = "red") +
facet_wrap("time", ncol = 4) +
theme_bw() +
theme(legend.position = "bottom")
```
### Time series and aggregations
Now, considering that we have a gridded dataset, we can compute zonal statistics per county.
In this example, we can analyse the temporal changes per county of the maximum precipitation.
We use the `stars::aggregate()` function, where we give an sf object to the `by` parameter that defines our zones, and a function to compute the aggregate statistic, in this case `max()`.
```{r}
precipitation_nc = aggregate(precipitation, by = nc, FUN = max)
```
We can plot this with base R and get an immediate glimpse of the result.
```{r}
#| fig-width: 8
#| fig-height: 7
plot(precipitation_nc, max.plot = 23, border = "grey",
lwd = 0.5, mfrow = c(5,5))
```
If we print this object you can notice that we no longer are working with a gridded data set (x/y are gone), but instead the dimension is the county geometry.
We have created now a vector data cube!
```{r}
precipitation_nc
```
We can transform this into a sf object to have another view of what we are dealing with.
```{r}
precipitation_nc_df = precipitation_nc |>
st_as_sf(long = TRUE) |>
st_join(nc)
precipitation_nc_df
```
With this we can create time series of maximum precipitation per county.
```{r}
#| code-fold: true
#| fig-width: 10
#| fig-height: 9
#| warning: false
ggplot(precipitation_nc_df) +
aes(
x = as.POSIXct(time),
y = test_stageiv_xyt.nc
) +
geom_point() + geom_line() +
scale_x_datetime(date_breaks = "12 hours", date_labels = "%H:%M") +
theme(legend.position = "none") +
labs(x = "Time", y = "Max. Precipitation (mm)") +
facet_wrap(~NAME)
```
Finally, to have a summary map of the maximum precipitation, we can calculate when was the date of maximum precipitation per county.
For this, we apply a custom function `index_max` that gets the index of the maximum precipitation value, when all values are not NA.
```{r}
index_max = function(x) ifelse(all(is.na(x)), NA, which.max(x))
```
We apply this function to the geometry dimension, which translates into a reduction over time operation.
```{r}
precipitation_max = st_apply(precipitation_nc, "geom", index_max)
```
And lastly, we obtain the dimension value for the corresponding index we computed.
```{r}
precipitation_max$when = st_get_dimension_values(
precipitation_nc, "time")[precipitation_max$index_max]
```
Let's plot the results!
```{r}
#| fig-width: 10
#| fig-height: 5
plot(precipitation_max["when"], key.pos = 1,
main = "Time of maximum precipitation")
```
```{r}
#| include: false
# automatically create a bib database for R packages
knitr::write_bib(c(.packages()), "packages-raster.bib")
```